Trends and developments in the automation of heavy rail operations
Posted: 5 March 2020 | Rob Goverde | 2 comments
Can mainline and rail freight operations take advantage of the opportunities offered by Automatic Train Operation (ATO)? Rob Goverde, Professor of Railway Traffic Management & Operations and Director of the Digital Rail Traffic Lab at Delft University of Technology, explores.
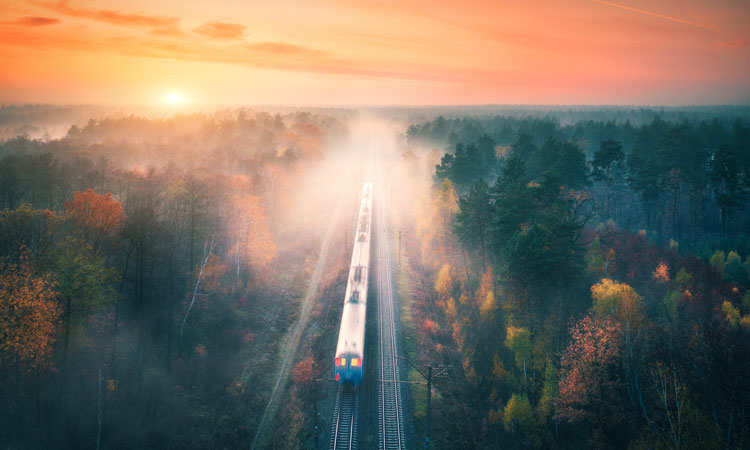
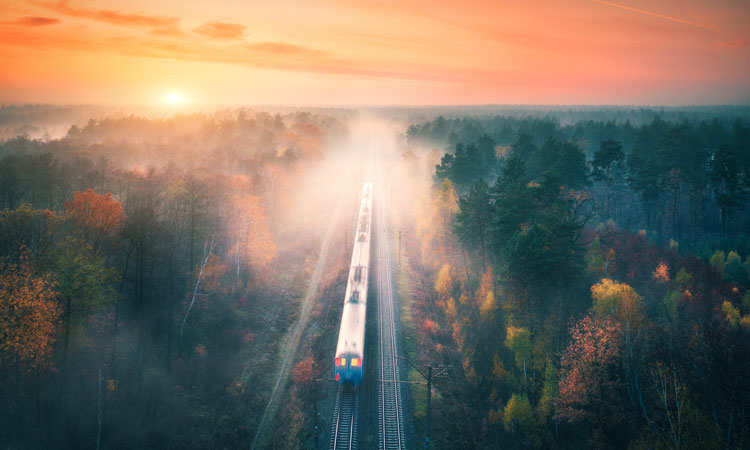
There have been many discussions recently about heavy rail becoming autonomously driven in the future. More and more metro systems all over the world operate automatically up to the highest Grade of Automation (GoA4) of unattended train operation. In contrast, heavy rail operation is only at the beginning of Automatic Train Operation (ATO).
In Australia, the Rio Tinto iron ore railways were fully automated recently, allowing trains to operate with GoA4. The Czech railways have been operating with GoA2 semi-automatic train operation (STO) since 1991 and, in the UK, the Thameslink has been operating with STO over ETCS Level 2 since 2019, with plans to upgrade to GoA4.
Other countries have initial ATO pilots, such as the Netherlands, which have recently carried out several GoA2 tests on both freight and passenger trains. Mainline railways have been more active recently in implementing Driver Advisory Systems (DAS) to support drivers with manual train operation (GoA1). Clearly, heavy rail operates in more complex environments than metro systems, which makes autonomous operation way more challenging. In particular, mainline railways are typically characterised by a variety of train types and stop patterns, long distances in open environments, complex station layouts with many conflicting movements, and operation by multiple railway undertakings, including passenger and freight.
What is the future of automation for heavy rail?
The goal of automation of railway operations is to improve capacity, reliability, energy efficiency, flexibility, safety, and cost effectiveness. Many railways experience an increasing transport demand, resulting in operation close to the infrastructure capacity limits. In such conditions, delays easily spread throughout the network. It is, therefore, of primary importance to avoid train delays and act effectively when they do occur. The main functions of ATO are accurate driving and accurate stopping, which implies better schedule adherence. As a result, the buffer time between trains can be reduced, allowing more trains on the network. However, the inherent variation in operational conditions – such as passenger volumes, rolling stock characteristics and weather conditions – cannot be ignored. The ATO algorithms, therefore, must be robust to daily variations and small disturbances. Nevertheless, it is unavoidable that schedule deviations will exceed the (small) buffer times once in a while, so ATO must receive up-to-date journey profiles to avoid conflicts. Therefore, ATO needs to be connected to a Traffic Management System (TMS) that monitors the train movements and is capable of conflict detection and resolution to maintain overall conflict-free timetables for conflict-free following, merging and crossing. In general, train operation is part of a railway traffic system with many interactions and feedback loops.
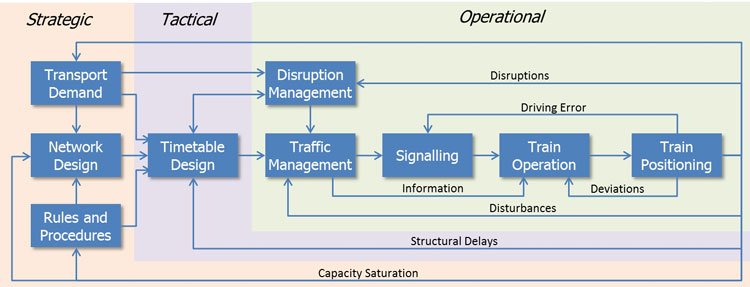
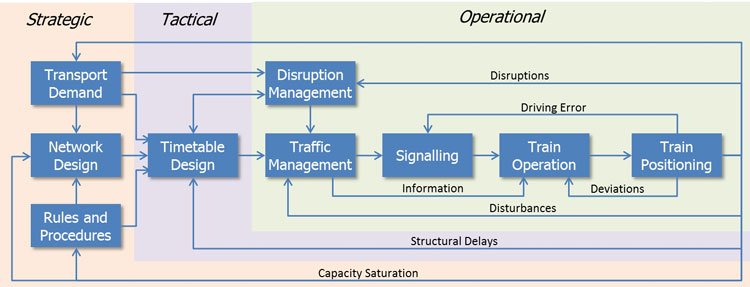
Figure 1: Railway traffic system
The automation of heavy rail operations, therefore, goes beyond automatic train driving. The EU project ON-TIME investigated what is needed to implement algorithms for automated timetabling, traffic management and connected DAS. The project ran between 2011 and 2014. At that time, ATO for heavy rail was still unthinkable and the focus was on DAS, but the developed principles of centrally guided train operation also apply to ATO. Automated railway operations should start with a conflict-free timetable computed at a microscopic level with drivable speed profiles. This timetable is the basis of a real-time traffic plan at route level maintained by a TMS equipped with automatic conflict detection and resolution algorithms. The TMS provides the ATO subsystems with up-to-date journey profiles with target times at timing points. The ATO system then generates a suitable speed trajectory and tracks it within certain tolerance bounds, while also sending timing point predictions back to the TMS for traffic monitoring. In parallel, the TMS feeds the real-time traffic plan to the automatic route setting systems so that consistent Movement Authorities are generated and sent to the Automatic Train Protection (ATP) systems, which provide the ATO on-board algorithms with safety constraints. ON-TIME developed a prototype systems architecture integrating all the developed algorithms and demonstrated its working in a simulation environment. One main lesson was, however, that the railways had difficulties in providing detailed infrastructure data to feed the algorithms. In the meantime, several initiatives have started to standardise infrastructure data and facilitate digital data exchange, such as the UIC RailTopoModel, RailML and the specifications of ATO-over-ETCS. This is an essential step in the automation of railway operations.
The goal of automation of railway operations is to improve capacity, reliability, energy efficiency, flexibility, safety, and cost effectiveness.
Real-time traffic management algorithms for conflict detection and resolution must still find their way to the industry, while microscopic timetabling methods are more and more adopted by various railways to develop accurate conflict-free timetables as a basis for reliable operations. Also, automatic generation of contingency plans to handle disruptions is being adopted by the railways, such as in the Netherlands, which is a first step to automatic real-time rescheduling algorithms for disruption management. In the meantime, the market for DAS is getting more mature with a trend to go from stand-alone DAS with advice based on a pre-programmed timetable to Connected DAS (C-DAS) that can receive timetable updates from a centralized TMS.
The performance of DAS and ATO relies on the method for speed trajectory generation. Simple ATO systems may consist of a cruise control where the cruise speed is predetermined by the timetable or may depend on the current delay. In the last decade, much research has been carried out on energy-efficient train driving. An energy-efficient speed trajectory makes optimal use of the running time supplements and track gradient profile by applying an optimal cruising speed, coasting regimes without traction and, possibly, regenerative braking. Advanced DAS and ATO speed trajectory algorithms compute the optimal switching points between successive driving regimes of acceleration, cruising, coasting and braking, depending on the timetable, current delay and the train and track characteristics. When trains operate fixed routes, the speed trajectories can be precomputed and stored on-board for online use. However, on mainline railways, trains may be rerouted or retimed, which requires a more flexible online computation of speed trajectories, depending on the current conditions. The future generation of C-DAS and ATO algorithms will thus contain online speed trajectory computations that can quickly adapt to changing conditions in interaction with a TMS.
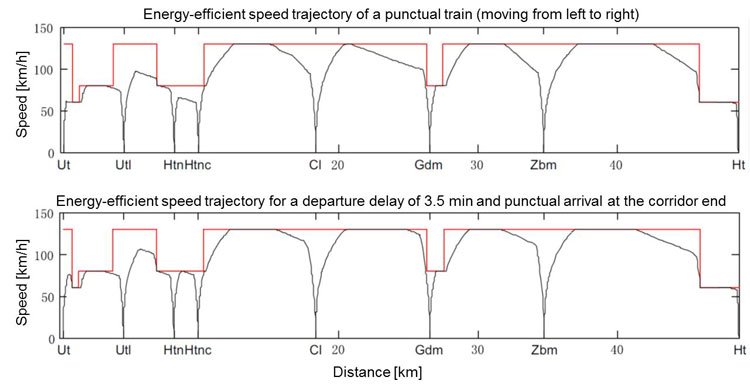
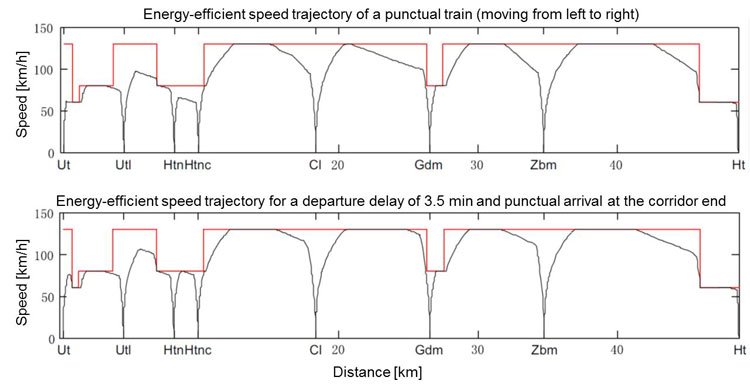
Figure 2: Speed trajectories
The train driver is responsible for the train operation in both GoA1 and GoA2. In GoA1, the train driver operates the train manually, supervised by the ATP and possibly supported by a DAS. While, in GoA2, the ATO directly commands the traction and braking controls after the departure command by the driver. The speed trajectory calculations may be the same, although manual driving may require restrictions on the amount of driver actions. In that sense, GoA2 means less workload, since the driving tasks are automated, although the driver may need to take over in case of failures or environmental conditions that the ATO system is not aware of.
For both DAS and ATO in GoA2, the human-machine interaction is essential. For DAS, a driver action needs to be announced well in advance so that the driver is prepared. Moreover, context information must be given so that the driver understands the advice and acts to it. Also, for GoA2 context, information must be given so that the driver understands what the ATO system is doing. This situational awareness is needed for the driver to carry out a supervisory role and be able to take over when needed. For driverless train operation (GoA3), automatic obstacle detection is required to automatically stop the train in dangerous situations, while staff in the train can still take over the driving in disruptive conditions. For unattended train operation (GoA4), the train may be remotely controlled from a traffic control centre in case of disruptions. GoA2 may be the highest achievable in open environments that restrict automatic obstacle detection. For high-speed and rail freight corridors, trackside fences may largely prevent obstacles moving on the tracks so that GoA3/4 is achievable. Other automation tasks are automatic turning and automatic shunting. These driving tasks apply typically to empty trains running at low speeds so that unattended train operation is feasible.
Artificial intelligence (AI) may improve automatic driving, stopping, traffic management and obstacle detection, both in terms of computational performance and learning from data. The recent Shift2Rail project, RAILS, is dedicated to investigating the potential of AI and develop a roadmap for AI integration in the railways. An important concept will be Explainable AI so that solutions can be understood by drivers and train controllers, as opposed to black box solutions. This is, at least, essential for GoA1 and GoA2, as argued above.
The future will bring even more automation to enable train-centric signalling systems, such as Virtual Coupling. Virtual Coupling is an innovative concept that allows trains to be ‘virtually coupled’ and operate cooperatively at relative braking distance using train-to-train communication. The potential of this concept is explored in the Shift2Rail project, MOVINGRAIL. Before such concepts can be realised, the railways will first take up advanced C-DAS and develop ATO GoA2, and then develop full automation on dedicated lines. It can be expected that the future heavy rail landscape will show a mixture of grades of automation, depending on the specifics of the railways.
Related topics
Automatic Train Operation/Autonomous Train Control (ATO/ATC), Cargo, Freight & Heavy-Haul, Digital Automatic Coupling (DAC), Digitalisation, Passenger Experience/Satisfaction, Regulation & Legislation, Technology & Software
Nice article, summarising the situation, describing the ATO system, and explaining its key benefits, professor Goverde! However, I do not agree with the following statement: “[…] between 2011 and 2014. At that time, ATO for heavy rail was still unthinkable […]”. For example, in the Czech Republic, we have ATO in GoA2 in operation for almost 30 years (since 1991). We have it on more than 300 vehicles and it can operate on a great number of lines – see e.g. the map here (red and orange lines): https://provoz.szdc.cz/PORTAL/Show.aspx?path=/Data/Mapy/ATO.pdf. We also tested the integration of AVV (i.e. the Czech ATO) system with ETCS on-board back in 2008 – see e.g. the video here: https://www.youtube.com/watch?v=9wt6JbHOVeU.
I think this article puts you in the position of an authority in the field of train operations.
Lately I also commented on ideas of Eelco Schrik (Mott McDonald).
I am currently involved in the European project FENIX.
Good luck and kind regards,