ABA measurements: Monitoring of Condition Based Maintenance during revenue operations
Posted: 21 May 2017 | Alfredo Núñez, Rolf Dollevoet, Zili Li | No comments yet
Rolf Dollevoet, Alfredo Núñez and Zili Li – Colleagues from the Section of Railway Engineering at Delft University of Technology – explore current developments in utilising passenger locomotives that use sensors to capture huge amounts of data to achieve monitoring and maintenance ‘during revenue operations’.
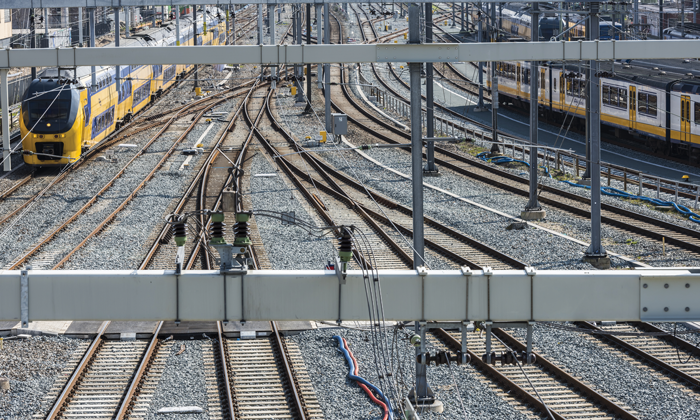
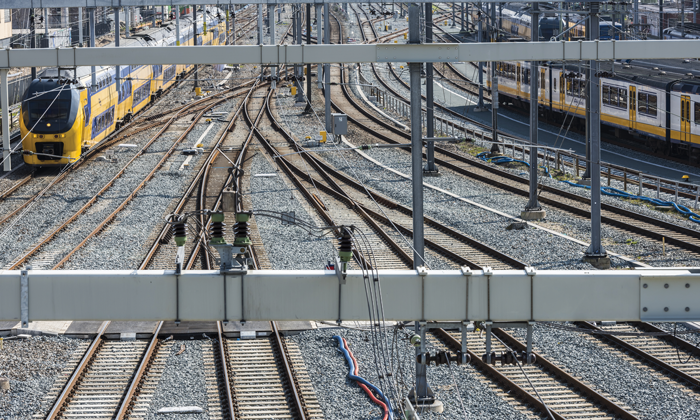
Today highly utilised railways in Europe are expected to provide services for passengers and freight almost 24/7. The increase in the availability of the infrastructure means that other track activities must take less time than they did before. Activities such as monitoring with specialised vehicles and maintenance are usually scheduled for night shifts or during period of time when the demand from elsewhere is less in order to avoid disruption. However, when unexpected situations occur in the infrastructure that compromise safety, it is usually inevitable that maintenance is performed as soon as possible and whenever needed. This often results in service delays and great annoyance to users. Even well planned maintenance operations at night might require extra time to finish properly due to unexpected situations in the field; and the consequence of this extra time is that the first and subsequent trains in the morning are delayed.
From a systems perspective, the goals are to enhance capacity, increase the reliability and quality of services, and reduce lifecycle costs. Under this high pressure, professionals in the railway industry must be prepared for a full ‘during revenue operation’ of both monitoring and maintenance. Monitoring the infrastructure from passenger trains in operation is a way to extend the classical concept of train service; not only as a way to transport people and goods, but to provide information about the condition of the infrastructure. Some technologies are currently ready for use in this way, and the example of axle box acceleration measurement will be discussed in this article. In the future, probably in tandem with advances in robotics, the same concept of ‘during revenue operation’ should be applied to the design of a whole new concept of trains that are able to perform maintenance actions such as grinding, tamping, ballast cleaning and renewal, fastening and railpads renewal, etc. The monitoring and maintenance during revenue operations bring many practical and scientific challenges involving multiple domains such as Railway Engineering, Electrical Engineering, Systems & Control, Computer Science, Big Data and Social Sciences, among others.
Big data paradigms in monitoring for Condition Based Maintenance
In the case of monitoring, recent advances in sensor technology for large-scale systems have enabled the acquisition of large amounts of data regarding the state of the infrastructure in railway systems during operation. For example; in the Netherlands the €2.4 billion ERTMS (European Railway Traffic Management System) project will provide many new opportunities for monitoring as well as new sources of data. However, in the railway industry there is still the need for a method to automatically convert data into actual knowledge; to find the best way to make use of all that mass of information – for instance, to provide input for theoretical models, and eventually to complement the monitoring strategy and maintenance decision making. Today, valuable information is essentially hidden behind a huge amount of terabytes measured by different sensors. Distributed sensors, both on-board measuring trains and at the side of tracks, are vital to measure and transmit the signals that are relevant to the detection of important changes in the infrastructure, its dynamics and variations. By using adaptive and intelligent signal processing methods, it is possible to extract the key information needed for the decision-making process to anticipate the impact of degradation and thereby determine the control measures needed to correct the problems in the infrastructure. Those control measures are incorporated in the contractor’s maintenance and planning list – i.e. the party that will ultimately implement the preventive and corrective actions to solve the problems in the physical system.
The railway data can be used for modelling, analysis, and supporting the decision-making of maintenance, yet follows a paradigm different from other traditional/old systems. From the theoretical and practical points-of-view, the utilisation of railway infrastructure information is challenging because it is multidimensional, spatially and temporally distributed, multiscale, and comes from heterogeneous data sources (vibration, video, sound, eddy current, ultrasonic, performance reports written by hand, etc.). Moreover, the data is influenced by specific external factors such as environment, characteristics of the actuators, errors from the sensors themselves, inaccuracies from collection by humans, incompleteness, etc., all of which affect the ability of conventional methodologies to manage key variables and compromise the performance of decision support systems.
Big data is a trend in diverse fields. In the past it referred to problems related to the processing of many megabytes of information, often regarding what is termed the ‘V’ of ‘Volume’. Nowadays, with the new capacity of computers, this ‘V’ of Volume is only one of the challenges, but other ‘Vs’ such as the ‘Variety’ of the data also come into play. Considerations such as how to deal with the coherent fusion of multiple sources of information; and how fast the whole process from data collection to actual knowledge should be (‘Velocity’) are under debate. ‘Visualisation’, ‘Veracity’, ‘Value’ of the data and its ‘Vulnerability’ are among other ‘Vs’. In the case of railways, the data can only become useful information when the proper unique characteristics of the physical components in railways systems are explicitly included (i.e. data veracity). The full value of the data can be obtained when its monitoring plays an important role in maintenance support decision systems, whose final decisions are implemented automatically or manually by operators (decision agents), based on the collected data (automatic or manual collection of information) in conjunction with their own experience of the system (knowledge sources).
Axle Box Acceleration measurements
In Dutch railways, Axle Box Acceleration (ABA) measurement has been used for the detection of squats1-4. An example of the data for a moderate squat is shown in Figure 1. The system has also been used to understand the degradation of insulated rail joints5,6. Figure 2 shows an example of the data of two insulated joints – one with light degradation and the other with a clearer plastic deformation. Other extensions include the detection of short pitch corrugation, the study of switches and crossings, transition zones and welds, among others.
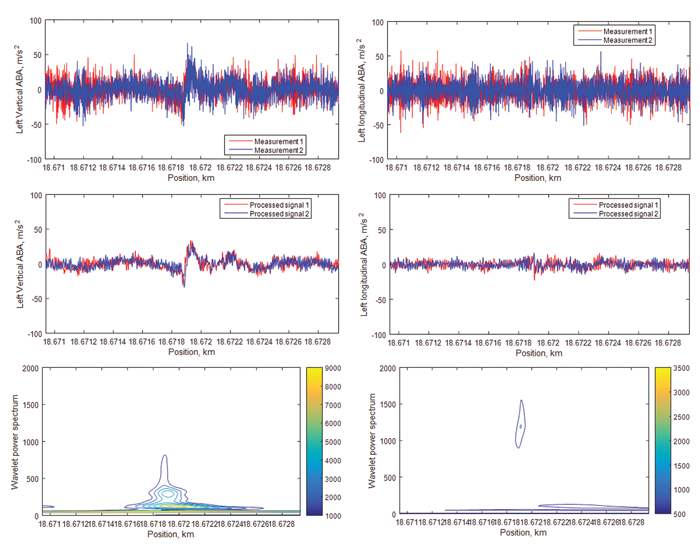
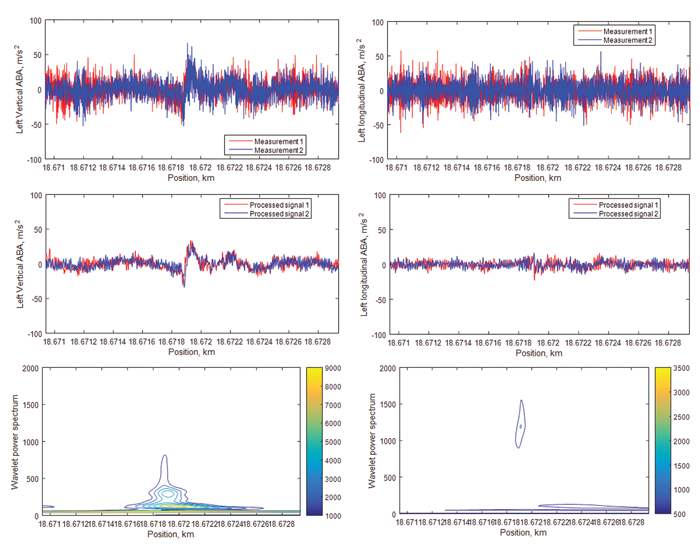
Figure 1: Example of measurements for a single moderate squat defect
The data is collected from accelerometers, a GPS receiver, and either a tacho or speed-sensor for positioning. For the monitoring of the entire Dutch railway (more than 6,500km of tracks), the measurement provides a data volume of several terabytes. To ensure veracity, multiple sensors and multiple passages are considered. The value of the measurement is twofold: the first is its lower costs once implemented in the operation (no need to book expensive trains for the daily monitoring of defects); and the second is that the information acquired under actual operating conditions can provide a more accurate indication of the effective severity of the defect. Rail defects can be viewed like an iceberg: the top is what is visible from systems such as video images, and the iceberg below the water surface is all the information that is not possible to see but that ABA can capture.
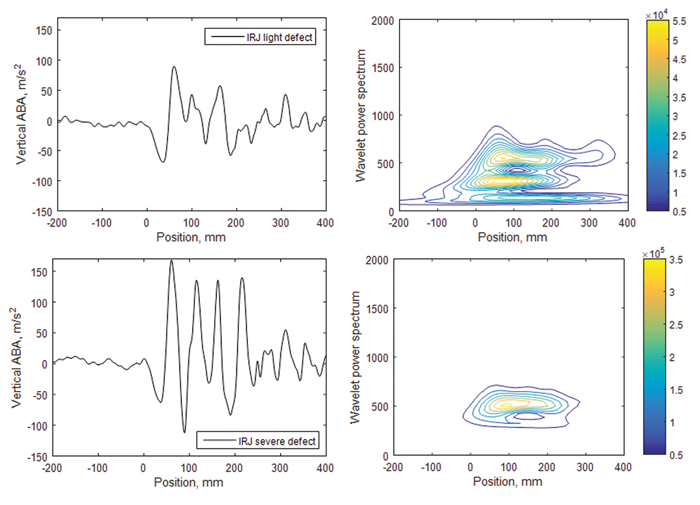
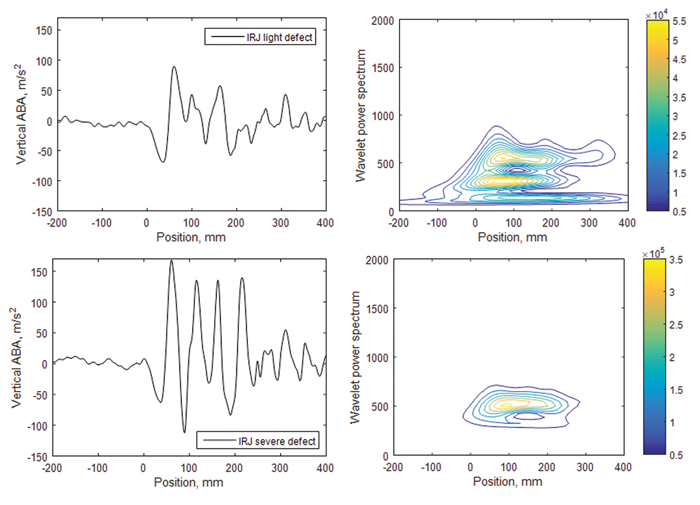
Figure 2: Example of measurements for two insulated rail joints: one with a light plastic
deformation and the other with a clearer plastic deformation
The system has been tested in a tram in Sheffield7. In 2016, within the framework of the H2020 project, NeTIRail-INFRA, ABA measurements were performed in the track near Brasov in Romania (as shown in Figure 3). Nowadays, frequent monitoring using the CTO Train of the Section of Railway Engineering is performed on the Dutch tracks (see Figure 4). From December 2016 to August 2017, every two weeks, the condition of the rail welds on the Dutch bridge will be monitored. Additionally, ProRail has asked to monitor more than 1,200km using the ABA system to explore real preventive maintenance together with contractors.
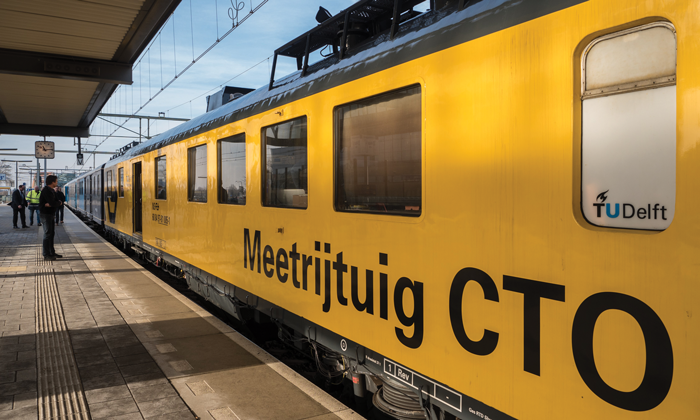
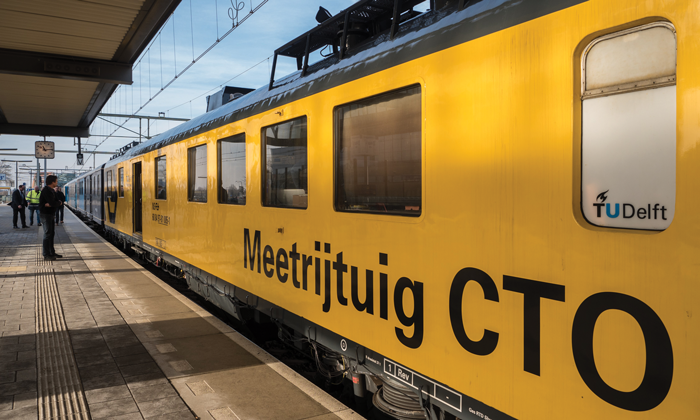
Figure 3: The measurement train of the Section of Railway Engineering at Delft University of Technology
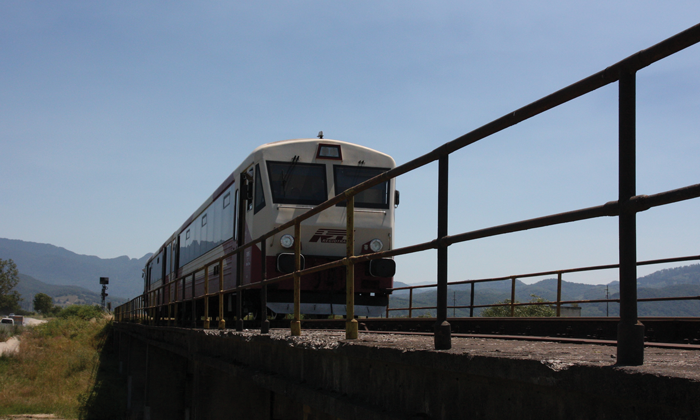
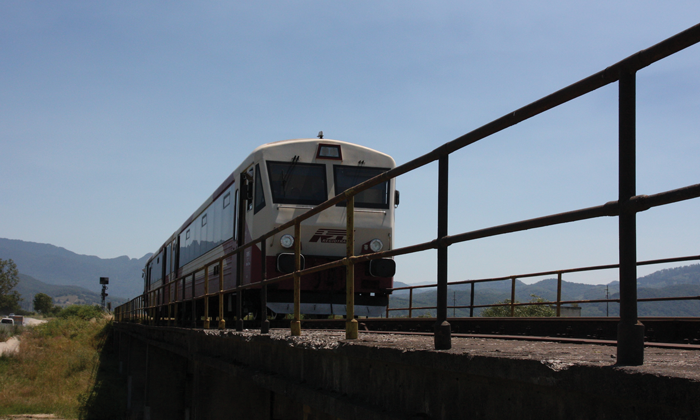
Figure 4: Passenger train in Romania; ABA measurements were conducted for the H2020 Project NeTIRail-INFRA
References
1. M. Molodova, Z. Li, and R. Dollevoet, “Axle box acceleration: Measurement and simulation for detection of short track defects”. Wear, Volume 271, Issues 1–2, 18 May 2011, Pages 349-356, DOI:10.1016/j.wear.2010.10.003
2. Z. Li, R. Dollevoet, M. Molodova, and X. Zhao. “Squat growth—Some observations and the validation of numerical predictions”. Wear, Volume 271, Issues 1–2, 18 May 2011, Pages 148-157, DOI:10.1016/j.wear.2010.10.051
3. M. Molodova, Z. Li, A. Núñez, and R. Dollevoet, “Automatic detection of squats in the railway infrastructures”. IEEE Transactions on Intelligent Transportation Systems. Volume 15, Issue 5, October 2014, Pages: 1980-1990. DOI: 10.1109/ TITS.2014.2307955
4. Z. Li, M. Molodova, A. Núñez, and R. Dollevoet, “Improvements in axle box acceleration measurements for the detection of light squats in railway infrastructure”. IEEE Transactions on Industrial Electronics. Volume 62, Issue 7, July 2015, Pages: 4385-4397. DOI:10.1109/TIE.2015.2389761
5. M. Oregui, M. Molodova, A. Núñez, R. Dollevoet, and Z. Li, “Experimental investigation into the condition of insulated rail joints by impact excitation”. Experimental Mechanics. Volume 55, Issue 9, November 2015, Pages: 1597-1612. DOI: 10.1007/s11340-015-0048-7
6. M. Molodova, M. Oregui, A. Núñez, Z. Li, and R. Dollevoet, “Health condition monitoring of insulated joints based on axle box acceleration measurements”. Engineering Structures, Volume 123, Issue 2016, September 2016, Pages: 225-235. DOI: 10.1016/j.engstruct.2016.05.018
7. M. Oregui, S. Li, A. Núñez, Z. Li, R. Carroll, and R. Dollevoet, “Monitoring bolt tightness of rail joints using axle box acceleration measurements”. Structural Control and Health Monitoring, 24:e1848, 2017. DOI: 10.1002/stc.1848