Birmingham Centre for Rail Research and Education hits half a century
Posted: 12 July 2024 | Dr Joe Preece - Birmingham Centre for Railway Research | No comments yet
For Global Railway Review’s latest Track Insight: Talent, Dr Joe Preece, Research Fellow at the Birmingham Centre for Railway Research and Education, shares insights on the transformative potential of artificial intelligence and the importance of data readiness in revolutionising the rail industry.
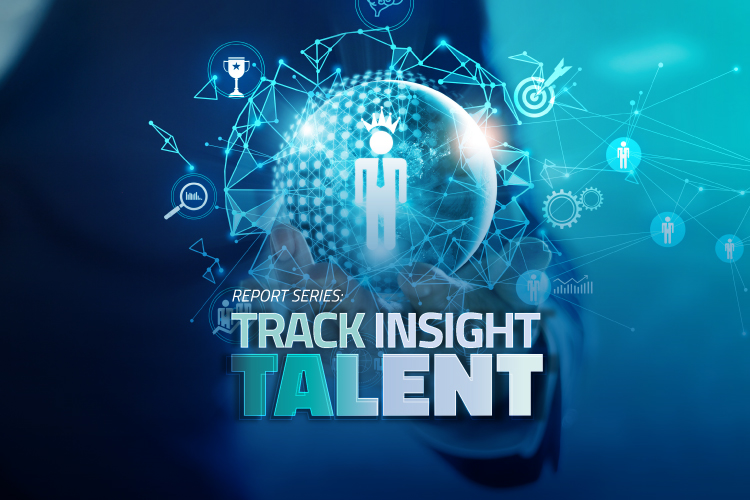
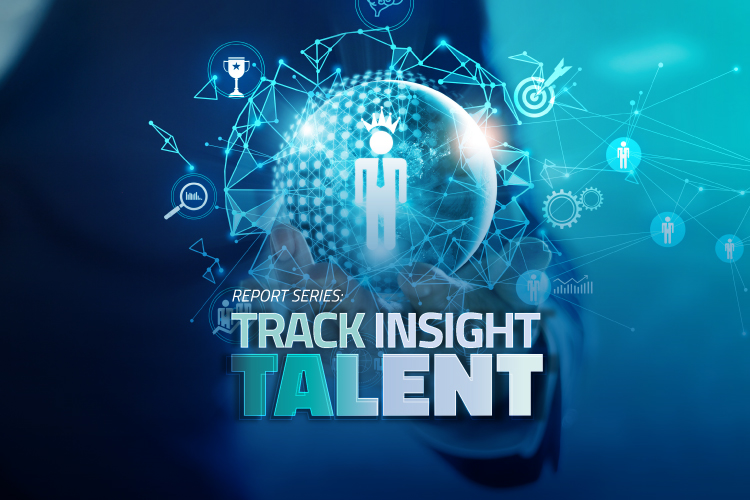
The Birmingham Centre for Rail Research and Education (BCRRE) – Europe’s largest rail research centre – recently celebrated its 50 years of pioneering research. It is fair to say that within this time, the department has witnessed a breadth of data-centric projects, driving advancements in rail both technology and infrastructure. Now, with technology evolving ever more quickly, new applications promise to revolutionise the rail industry in a new age of artificial intelligence (AI).
BCRRE recently partnered with Network Rail to utilise data from their New Measurement Train (NMT), in order to build ML models to predict Track Quality Index (TQI) degradation rates”
Asset maintenance is one such area that will benefit from fresh perspectives, thanks to advancements in machine learning (ML). BCRRE recently partnered with Network Rail to utilise data from their New Measurement Train (NMT), in order to build ML models to predict Track Quality Index (TQI) degradation rates. Ultimately, these models will enable asset owners to accurately forecast failures, and – partnered with additional models to optimise maintenance schedules – could increase efficiency while reducing costs. This innovative approach is also being applied with MoniRail, a spin-out company of BCRRE. MoniRail installs in-service condition monitoring equipment with the potential to capture critical data, further enhancing the accuracy and efficiency of predictive maintenance in the rail industry.
Other research explored how Distributed Ledger Technologies (DLTs) are paving the way for smarter ticketing systems. One major issue that plagues current systems is the lack of interoperability between them. The System for Ticketing Ubiquity with Blockchain (STUB) addresses this by using DLT to ensure that ticketing data is accessible and traceable, with security baked-in. This technology is designed not to overhaul all existing systems, but to supplement them with a core data architecture capable of sharing the most important assets; the tickets. By opening up ticketing data, STUB has the potential to significantly improve multi-modal and cross-continental journeys, making travel more seamless and efficient for passengers.
Furthermore, we are using innovative applications of computer vision and point clouds are transforming accessibility within railway stations, having developed a routing engine aimed at providing tailored guidance for passengers. This advanced system is designed to avoid crowds and offers suitable routes based on the passenger’s specific needs, such as avoiding stairs for wheelchair users. By making stations more navigable and accessible, this technology greatly enhances the travel experience for all users, ensuring smoother and more efficient journeys.
AI in rail: Transformative potential and challenges
By opening up ticketing data, STUB has the potential to significantly improve multi-modal and cross-continental journeys”
Despite the excitement surrounding AI, the question of industry readiness to use such technologies remains prevalent in my mind. In practice, and particularly in complex business environments, data cleanliness and availability often pose significant barriers. The rail industry does not readily incentivise data sharing or uphold robust data hygiene practices, greatly hindering the potential of cutting-edge AI technologies, and we must therefore remain pragmatic in creating tangible solutions to address existing challenges. Ensuring proper data management and fostering a culture of sharing are critical steps towards fully realising the transformative potential of AI in rail.
Of course, this is easier said than done. But if we cast our minds forward by a decade, we can begin to imagine how AI might be able to address these issues. For example, imagine a platform capable of ingesting data, automatically classifying it, and extracting metadata, all while linking it with other datasets. This decentralised approach ensures data ownership and storage remain with the original holders. Using federated machine learning, new insights can be built from other datasets that may not be directly accessible. Large language models (LLMs) allow for more human-centric interactions with the system. An embedded economy lets users buy and sell data by usage, with each transaction traced and provenance assured. While these ambitions are still speculative, the rapid growth of technology suggests we may be closer to achieving them than we think, and to me, this is the direction we must head in.
There is much to be done in the rail industry data ecosystem, but AI may hold the key to solving many of these challenges. If we get this right now, we can fully utilise AI across vast amounts of collective data, fostering a competitive yet open ecosystem involving industry, government, and academia. By addressing data readiness and incentivising sharing, we can unlock the full potential of AI, driving innovation and efficiency in the rail industry.
Related topics
Digitalisation, Global Railways Review's Track Insight: Talent, Safety