Should Algorithmic Decision-Making (ADM) in railways be regulated?
Posted: 14 April 2023 | Maria Price | No comments yet
Maria Price examines the complexities of regulating Algorithmic Decision-Making (ADM) in railways with differing expectations depending on the subject (human or non-human) whose data is processed.
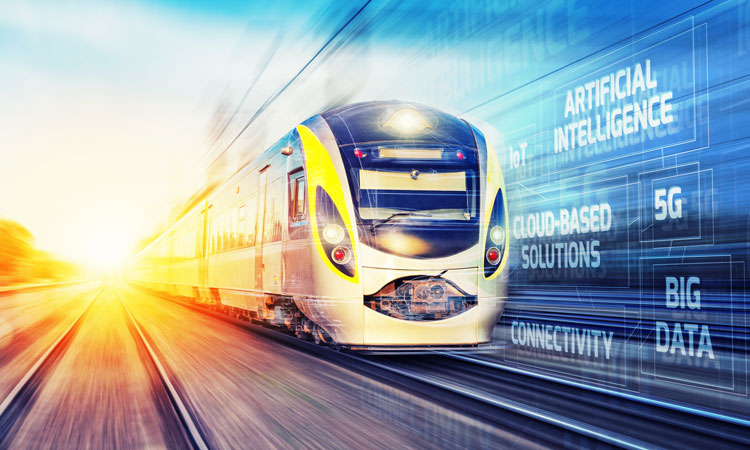
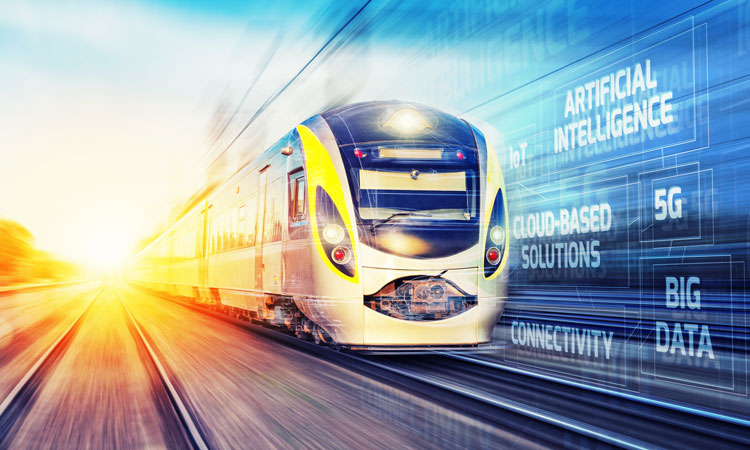
Algorithmic Decision-Making (ADM) is the ability to process and analyse large amounts of data to obtain certain information, to identify patterns and correlations, to make recommendations, or to trigger events. Algorithms are developed to train data to assist or substitute human decision-making. When integrated with machine-learning capabilities, it can monitor, predict and provide alternative solutions. ADM with machine-learning capabilities can be particularly relevant and useful in the development of sensors and software products for the management of railway assets, automation of operations and assisting customers when using travel platforms such as journey planners or e-ticketing apps.
ADM can be code-based (rule based) or data-driven (Hildebrandt, 2018). One example for code-based decision-making in railway operations is the use of sensors that are programmed to identify smoke, such as cigarettes in train lavatories. Once the sensor identifies the smoke, it would trigger an alarm and stop the train. These sensors would be coded on the principle of ‘if this, then that’.
Data-driven decision-making is more complex as it would require advanced techniques for the processing of the collected data. One example is the use of journey planners where the tool has the machine-learning capabilities to learn about the passenger’s travel behaviour and recommend the best connections, best routes and possibly offer travel deals.
…ADM could help train drivers to monitor the route and the performance of the train, recognise any anomalies and prevent potential derailment.
ADM can be fully automated, or it can also have human intervention. In the case of the cigarette smoke in the train, the ADM is limited to the braking of the vehicle. As a next step, the train manager and the driver have a series of radio exchanges, where additional checks are made before the re-departure of the train.
ADM systems are becoming popular and are already implemented in many sectors and industries, such as healthcare, banking and marketing. A study by the European Parliamentary Research Services has pointed out the possible risks of misuse of algorithmic decision systems (ADS), which may lead to biases, discrimination or unfair practices, which can impact not only people and society, but also technology and markets (EPRS, 2019). The European Union (EU) has worked hard to introduce a digital strategy and legislations such as the Digital Markets Act, the Data Governance Act, the Data Act, the Digital Services Act and the General Data Protection Regulation (GDPR), to name a few. These legislative initiatives focus on processes and structures to share data among entities, persons and the public sector, introduces measures to access, use and transfer data, and defines rights and responsibilities in the use of personal data.
If there are risks and challenges associated with ADM in railways, do the abovementioned legislative texts sufficiently regulate or overregulate these systems? The answer depends on who is being asked: i.e. the consumer, the user, the manufacturer, a railway company or an authority. To follow are several examples, where depending on the subject (human or non-human) whose data is processed, there would be different expectations for regulating ADM.
Operation of trains
The first example is the operation of trains, where ADM could help train drivers to monitor the route and the performance of the train, recognise any anomalies and prevent potential derailment. In such cases, the ADM could play a preventative or ex-ante role and assist the driver in ensuring the safe operation of the train. Technologies such as derailment detection devices (DDD) collect data that monitors the behaviour of each vehicle on the train, the condition of the infrastructure, the speed, the curve and other factors in order to determine the potential risks for derailment.
…there is growing interest for wagon keepers to install monitoring and tracking devices on their freight wagons so that they can monitor the performance and the condition of the fleet to determine when a wagon is damaged, or is in need of maintenance and repair.
These sensors would calculate the data in a way that they can assess the risks. They are integrated with machine-learning and analytics capabilities making it possible to trigger a message or an action, in which case automatic braking would activate to slow down the train. During this process, the driver of the locomotive would receive a message in the cabin, and they would be able to decide to override the braking, or to let the locomotive automatically reduce the speed as determined by the sensors. This is an example of positive ADM. However, there could be a risk that with such an automated decision, the driver may put too much reliance on the DDD sensors and rely less on their own experience as a driver. A derailment might still occur due to technical failure, miscalculation, or even sabotage of the sensor. Who would be accountable, responsible and liable in this case? The driver, who relied on the ADM of the device, or the company whose codes failed to process and train the data to calculate correctly the algorithm? Both, the safety and the integrity of the data has been compromised, leading to the wrong consequences.
Wagon keepers
In the second railway example, there is growing interest for wagon keepers to install monitoring and tracking devices on their freight wagons so that they can monitor the performance and the condition of the fleet to determine when a wagon is damaged, or is in need of maintenance and repair. Mileage data is also crucial for maintenance intervals as well as determining the leasing costs. The wagon keepers are companies that own, manage and lease freight wagons to railway companies to use them to transport goods nationally and internationally. The keepers have a contractual arrangement with the railway operators or railway undertakings for the use of these wagons. While the keeper sees a benefit in installing GPS, sensors and other measuring devices, the railway companies might be less supportive because that would mean that there would be data and information on the vehicle status, which might affect their commercial interests and competitiveness. Current railway legislation does not regulate data management and interfaces with railway contract law. There are general principles of contract law for the use of wagons in the form of Uniform Rules concerning ‘Contracts of use of vehicles in international rail traffic’ (CUV) and ‘General Contract of Use’ (GCU), but there are no provisions, nor soft law explaining how and how much of the collected data should be managed.
Passenger rail transport
The third example transitions from freight rail transport to passenger rail transport, where risks and challenges could be identified in the use of ADM in the electronic purchasing of tickets and digital journey planning. In Europe, there is a strong policy goal to have a harmonised approach to e-ticketing to facilitate passenger mobility and improve their travel experience. Passengers are already able to use apps or websites to organise their trips by train around Europe. Mobile ticket purchasing sometimes (if not usually) asks for location data to be switched on in order to provide the passenger with the best connection from the nearest station or with real-time travel information. Combined with Google maps, a person would be surprised to see that at the end of the day they could get a full report of the trip, the type of transport used, kilometres passed, and even the last time the person was in that area. The app would record the selected stops during the journeys, and they would pop up automatically when a person plans to travel again.
…risks and challenges could be identified in the use of ADM in the electronic purchasing of tickets and digital journey planning.
On one side, this machine-learning capability can be seen as positive innovation, because it would speed up the booking process and passengers would not be required to repeatedly enter the same data. While this is seen as a travel convenience, it is a form of monitoring the movement of the passengers. Having the location always activated can be argued as an infringement of privacy and a form of ‘commercial stalking’. The mobility app also has credit card information, personal details and billing information. Although assured that the app is reliable, it is not known how this information is stored, kept secure, if it is used for statistics and analyses or shared with third parties. The potential problem of the ‘black box’ where the user of the app is not aware of the way the travel app processes his or her data could arise. The paradox in this case, is that the benefits outweigh the possible risks and there are not known cases where users of travel apps complained about the lack of transparency or that their data was abused. The greater risk is probably the security of the passenger data.
Ensure transparency and accountability
ADM is continuously evolving and becoming more intelligent in its capabilities.
These three examples show that to reduce scepticism and mistrust, we need to assess the regulatory approach that ensures transparency and accountability when ADM is applied in daily operations. Should ADM systems and their applications be regulated through international standards, or would it be sufficient to establish contractual agreements between data providers, data controllers, data hosts and tool suppliers? How would ADM systems be regulated if they process data related to cross-border operations. Would this be a matter in the scope of international convention, or a legal framework at national and regional level?
The use of sensors, tools and solutions such as mobile monitoring, scanning and automated testing of vehicles can help collect data fast, analyse it and assist the staff responsible to make decisions on the readiness of the vehicle and the train. Such data is not human sensitive; therefore, regulating it at contractual level would be sufficient.
The questions of ethics and human impact arise when we speak of passenger related data, or data related to medical checks, competences and skills of train drivers and train staff to operate trains nationally and internationally. Although there is an EU Train Drivers Directive and operational rules in place, it could affect national requirements and regulations on the management of such private (or identifiable) data across sectors, not just the railways.
A co-regulation approach?
…depending on the business case and how ADM is used in fleet management, train operations, maintenance and travel planning, a co-regulation approach to regulating ADM might be most appropriate.
ADM is continuously evolving and becoming more intelligent in its capabilities. Therefore, it would not be possible to fully regulate the system, the software, its application and to control all potential risks. Some new risks could arise following deployment and experiences. Therefore, depending on the business case and how ADM is used in fleet management, train operations, maintenance and travel planning, a co-regulation approach to regulating ADM might be most appropriate. Co-regulation would mean a regulatory framework presenting the overall principles, high-level requirements and the roles and responsibilities to gather, manage and share information, combined with the autonomy of the sector to develop and implement common standards and processes for the application of ADMs. In this case, the regulatory framework should be seen as an enabler to the development and implementation of such innovative solutions while safeguarding ethics, accountability and transparency.
References:
- Hildebrandt, M. 2018. Algorithmic regulation and the rule of law. Philosophical Transactions of the Royal Society A: Mathematical Physical and Engineering Sciences. 376 (2028):1-11.
- European Parliamentary Research Service Scientific Foresight Unit (STOA). 2019. Understanding algorithmic decision-making: Opportunities and challenges STUDY Panel for the Future of Science and Technology. PE 624.261. Available: https://www.europarl.europa.eu/RegData/etudes/STUD/2019/624261/EPRS_STU(2019)624261_EN.pdf
Related topics
Big Data, Cargo, Freight & Heavy-Haul, Digitalisation, Regulation & Legislation
Related organisations
Intergovernmental Organisation for International Carriage by Rail (OTIF)