Robotics and industrial AI for track maintenance
Posted: 7 January 2021 | Diego Galar, Uday Kumar | No comments yet
Diego Galar and Uday Kumar, Professors at Luleå University of Technology, explore how more sophisticated inspection, condition monitoring, analysis and maintenance technologies can facilitate cost effective and efficient rail asset management.
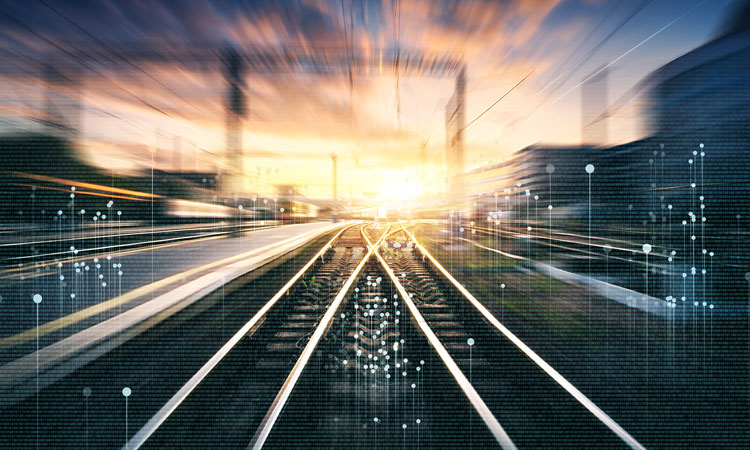
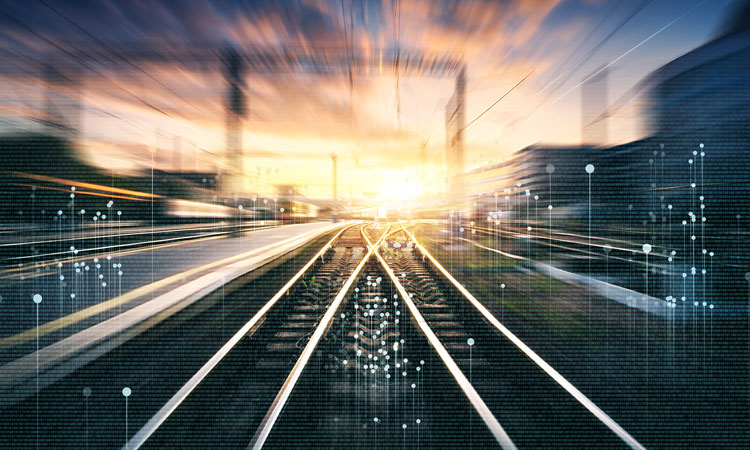
Rail as a linear asset
A track is an engineering infrastructure that usually spans long distances and can be divided into segments, all of which perform the same function but may be subject to different loads and environmental conditions. Conventional asset management systems or decision support tools are not suitable for linear assets, as they have a hierarchical structure based on parent–child relationships. In contrast, linear assets have linear properties – for instance, similar underlying geometry and characteristics – over a distance. They show specific patterns of continuous inherent deteriorations and failures. Therefore, remedial inspection and maintenance actions will be similar along the length of a linear asset, but, because the asset is distributed over a large area, the execution costs are greater.
Various types of engineering systems have been developed to acquire data in an automatic way during inspections or to perform maintenance tasks. These are mostly semi-automated systems, i.e., robotic applications. The fields of application range from welding rails to inspecting steel bridges. In general, robots need to be developed for specific tasks and fields of application, as these define the suitable locomotion principles or adhesion systems and the robot’s dimensions.
Challenges in inspection and maintenance
Industrial maintenance service providers find that creating a railway taxonomy to accurately represent linear rail assets is a highly complex process, making maintenance and inspection strategies difficult to plan and report. Yet, maintaining the integrity of these assets ensures performance optimisation and compliance with health safety and environmental (HSE) regulations.
Managing linear assets, such as rail networks, comes with a unique set of requirements. Asset management functionality is associated with conducting detailed inspections, planning maintenance schedules, devising overhaul schedules and prioritising work to meet safety, budget and customer expectations. To maintain linear assets, asset managers must do the following:
- Split assets into sections based on a definable length breakdown
- Add records for associated assets along lengths, such as locks, gates, footpaths etc.
- Record multiple ownership records for single assets
- Import historical maintenance records, images and any additional data in a dynamic manner
- Perform on-site, roaming inspections using autonomous devices to record defects, identify issues, add images, log GPS data and add any other information required
- Perform maintenance activities through a remotely operated interconnected environment.
Once linear asset management in railways is properly performed and infrastructure managers have asset registers and other relevant tools, robotics have value. Various different remotely controlled and autonomous inspection and maintenance devices can be used on railways for a number of different purposes, including:
- Identification of obstacles and track irregularities using drones
- Inspection of rail profile, cracks, irregularities and missing components using autonomous robot vehicles
- Replacement of missing components, crack welding, etc. using autonomous maintenance robot vehicles.
Smarter drones for rail inspection
Unmanned aerial vehicles (UAVs) have attractive features for inspection purposes, such as flexibility, adaptability and a range of payloads. Sensors include high-resolution digital and infrared cameras, light detection and ranging (LiDAR), geographic information systems (GIS), sonar sensors and ultrasonic sensors; most can be adapted to a UAV platform. A close-up photograph of a bridge structure or tunnel, difficult for inspectors to reach, will show maintenance personnel potential defects and degradation as a valuable input for maintenance planning and also for train operation. For catenary and other feeding elements, drones equipped with forward-looking infrared (FLIR) or ultraviolet sensors can detect hot spots or corona discharge on conductors and insulators, signalling a potential defect or weakness in the component.
However, industrial artificial intelligence (AI) and deep learning are an important part of the inspection process because of the different nature and granularity of data in the form of pictures, videos and other readings.
At present, UAVs are remotely operated; the next phase of UAV technology will be to deploy machines that can fly autonomously. This technology will allow UAVs to sense and avoid other objects in their path, recognise features or components through various sensors (including cameras) using complex software algorithms, such as image processing algorithms, and achieve situational awareness. This advanced technology will foster calculated decision making, such as initiating focused inspections, issuing work orders for repairs and starting maintenance work with the same robot or another autonomous robot integrated in the system.
Autonomous robots
Many different robots have been developed to handle various situations on railway tracks, but most are limited to special situations or applications. To execute the desired tasks, autonomous robots, as well as all other technical systems, must fulfil certain requirements. The requirements and their importance and focus depend on the individual application or tasks.
To be able to scale up and generalise the use of autonomous vehicles, a dynamic asset maintenance and management plan must be deployed with the help of AI technologies and analytics. Right now, industries are using the devices separately for inspection and maintenance; the two have not yet been integrated. By integrating the two operations with the available ICT technologies, the asset maintenance and management process can be automated. Moreover, the incorporation of AI together with cognitive analytics tools can make the whole process dynamic and autonomous. However, maintenance decision support systems are not yet that mature in railways. We need a structured approach and concept to improve information extraction and knowledge discovery for optimised maintenance actions.
Maintenance analytics (MA) in railways aims to facilitate maintenance actions through the enhanced understanding of data and information. The railway system is complex and, depending on the element considered, the type of analytics will be different. There are four basic types of analytics:
- Maintenance descriptive analytics
- Maintenance diagnostic analytics
- Maintenance predictive analytics
- Maintenance prescriptive analytics.
Maintenance decision-making requires the ability to deal with faults (i.e., the inability to perform as required due to an internal state) and failures (i.e., the termination of the ability of an item to perform a required function). The relationship between faults and failures can be described as follows: A fault is the result (state) of an associated failure (event). Their analysis calls for time-ordered analytics.
Maintenance descriptive analytics
The descriptive phase of maintenance analytics aims to answer, ‘What happened?’. In this phase, it is very important to have access to data related to system operation, system condition and expected condition. To understand the relationship of events and states, it is important to understand the time and time frame associated with each specific event log. This means time synchronisation becomes an important part of maintenance analytics. In track maintenance, inspections of rail or singular elements with sensors, cameras or LiDAR are part of a process of ‘nowcasting’, where a problem is detected and eventually identified. Analytics focus on sensor readings and alarms.
Maintenance diagnostic analytics
The diagnostic phase of maintenance analytics aims to answer, ‘Why has something happened?’. The outcome from maintenance descriptive analytics is used to frame the diagnostic analytics. This phase requires reliability data, as well as the data used in the descriptive phase. When diagnostics can be applied to track maintenance, the root cause of the problem and not just the symptom can be removed; i.e., maintenance actions impact the initiator rather than simply mitigating undesired effects. For example, grinding or tamping based on track geometry without this type of analytics could cause over-maintenance that is harmful for the rail.
Maintenance predictive analytics
The predictive phase of maintenance analytics aims to answer, ‘What will happen in the future?’. This phase uses the outcome from maintenance descriptive analytics. Reliability data and maintainability data are required, along with the data used in the descriptive phase. In addition, to predict upcoming failures and faults, it is necessary to include business data, such as planned operation and planned maintenance. Past readings and data from sensors in real-time are crucial to estimate the remaining useful life (RUL) of the track or singular components. Prognosis is the most valuable information to optimise the maintenance schedule, but, unfortunately, limitations in the access to data or domain knowledge for degradation reduce the accuracy of predictions.
Maintenance prescriptive analytics
Track maintenance is opportunistic maintenance because of the unavailability of repair machines, such as tamping or grinding machines, since they are distributed and allocated where needed. This makes it crucial to decide what to do and when. The prescriptive phase of maintenance analytics aims to answer, ‘What needs to be done?’. The outcomes of maintenance diagnostic analytics and maintenance predictive analytics are both used in this phase. In addition, to predict upcoming failure and fault, there is a need for resource planning data and business data. Briefly stated, for track machines, different scenarios are considered as a function of the degradation and future operation to allocate resources in an optimum way.
Prescription is relevant but still requires a human to decide where resources should go.
The next step is cognitive analytics, where prescription and therefore maintenance actions are performed in an autonomous way. In fact, because of the size and complexity of the railway network, robotics for inspection and maintenance and cognitive analytics as the decision support system seem a feasible possibility.
Summary
Autonomous inspection and maintenance in railway linear assets is increasingly important because many of these assets are ageing – especially in Europe, where infrastructure is old and more capacity is demanded. At the same time, asset managers are struggling to operate effectively and maintain costs. Reliable inspection and maintenance methodologies incorporating new technologies – specifically, emerging ICT technologies – facilitate cost effective and efficient asset management. The industry movement towards new and more sophisticated inspection, condition monitoring, analysis and maintenance technologies, together with the development of autonomous robotics and cognitive analytics, will provide a platform to maintain railways more efficiently. The technology is available, but integration remains a key concern.
Issue
Related topics
Artificial Intelligence (AI), Digitalisation, Technology & Software, Track/Infrastructure Maintenance & Engineering