Improving rail crossing safety with artificial intelligence
Posted: 25 September 2020 | Karsten Oberle | No comments yet
Artificial intelligence (AI) and machine learning are increasingly being applied to some of the thorniest issues that rail operators confront, and one of the more promising ones is the monitoring of level crossings. Karsten Oberle, Head of Rail, Transportation Segment at Nokia Enterprise, explains more.
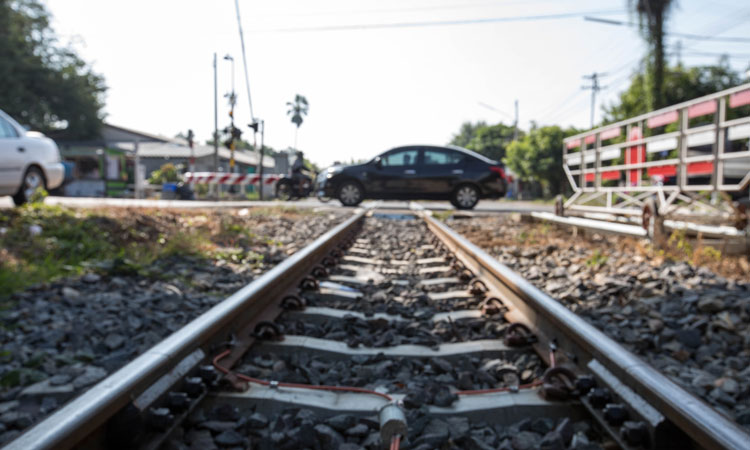
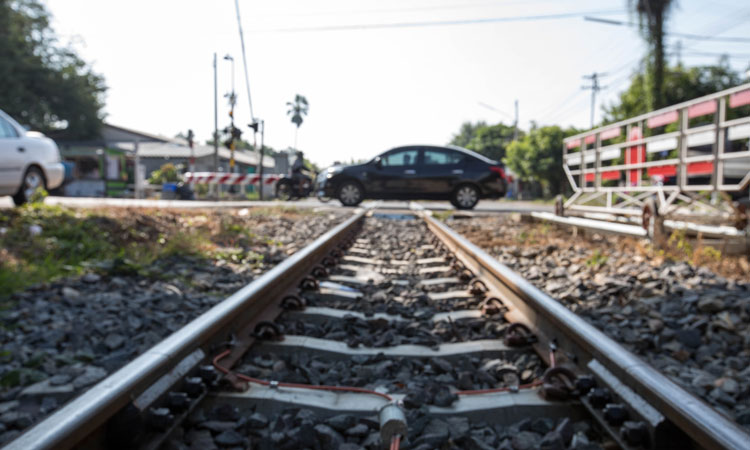
There are good reasons for the excitement around the application of video analytics, AI and machine learning to improve safety at level crossings. From 2009-2018, the U.S. Federal Rail Administration (FRA) reported an average of more than 900 injuries and 250 deaths at railway crossings annually. In the EU in 2018, Eurostat reported that there were 447 accidents at level crossings, which were also the cause of more than one in four of all railway fatalities.
For several years now, rail operators have used CCTV cameras to monitor level crossings and other parts of their infrastructure in order to reduce incidents and improve safety for the public and employees. The challenge with video, however, is that it often requires someone to continuously watch the feed in order to detect safety anomalies. Unsurprisingly, however, human operators can develop ‘video blindness’, and after relatively brief periods of time monitoring multiple video feeds, they can fail to see incidents that the cameras are recording.
A study by the Transportation Research Record in 2018 looked at the use of AI in video analytics for railways and found that it could significantly reduce the laborious effort needed to process video data from CCTVs and would reduce the effect of ‘video blindness’ on operators, thus improving their well-being and quality of work. Most importantly, it could correctly detect near-miss events associated with unsafe trespassing at railway crossings, which could lead in turn to the development of safer level crossing technologies.
A study by the Transportation Research Record in 2018 looked at the use of AI in video analytics for railways and found that it could significantly reduce the laborious effort needed to process video data from CCTVs and would reduce the effect of ‘video blindness’ on operators…
One of the reasons that the application of video analytics holds such promise is that CCTVs have been so widely adopted by the industry. In effect, video analytics has the potential to transform an almost universally available resource – installed CCTV cameras – and turn them into cutting-edge IoT sensors at a relatively low cost to operators.
The big step forward in the development of video analytics has been applying machine learning to detect these anomalies in the video stream without human supervision, and then alerting railway personnel to the possibility of an issue. In addition, some systems also feature object detection to recognise if the anomaly is a car or a person, which can help speed up safety precautions.
This new, more successful approach involves training the analytics software – the algorithm – to understand what an empty level crossing looks like, and then have it only react to changes against that known and invariable pattern.
The logic of this approach is much simpler. The analytics program can be instructed to ignore activity on the level crossing until the closing of the gates. At this point, the tracks should be empty.
Upon barrier closing, any changes to the digital video pattern of ‘empty tracks’ is then treated as an ‘event’, which can trigger an alert to a human operator to review the footage. When the operator reviews the anomalous video footage, they can then take action if it is required.
In this approach, the AI engine does not have to be as smart as a human, it just has to do the boring job of monitoring the 99.9 per cent of the video stream when nothing is actually happening. Over time and thousands of hours of analysing footage the algorithm can be trained to recognise patterns that don’t require an alert, such as rain or snow, leaves and other normal environmental events. Personnel can tag this kind of footage so that the software gets constant feedback to improve its abilities and reduce the number of false positives.
This kind of video analytics solution also simplifies the forensic analysis of incidents, including identifying near misses. The software can simply be instructed to discard or archive all footage that corresponds to the normal view of the scene, which not only drastically reduces the amount of video storage required, but also decreases the amount of footage that the forensic team has to review.
As such data is collected across hundreds of thousands of level crossings, it is not hard to imagine that the lessons learned will lead to much greater safety for the public and big operational savings to the rail operators. One operator that has already run trials on this type of enhanced railway crossing safety is Japanese rail operator Odakyu Electric Railway.
…using CCTV cameras to monitor railway crossings using machine learning isn’t simply a futuristic and unaffordable solution, but rather a practical way in which most rail operators could easily improve safety at railway crossings today.
With testing at the Tamagawa Gakuenmae No.8 railroad crossing in Machida City, Tokyo, Odakyu has been working to detect abnormal events by applying machine learning-based AI to available camera images.
Analysing available image feeds generated by conventional railroad crossing cameras, the software can identify potential issues in real time. Running on edge computing resources, it can also greatly reduce required bandwidth at remote sites, which may have limited connectivity.
The primary benefit of this kind of approach to video analytics is its ability to add an extra level of safety – in addition to existing deployed safety systems – by transmitting real-time alerts to external systems when important activity is detected. This enables the railway crossing sensors to integrate with existing operational processes, augmenting the knowledge and decisions made by security and safety personnel, without replacing them. This also helps with acceptance of the system by operations personnel.
Finally, because these systems can work with most existing IP cameras, and even older, lower resolution cameras, they don’t require an expensive upgrade to modern HD CCTV systems. This means that using CCTV cameras to monitor railway crossings using machine learning isn’t simply a futuristic and unaffordable solution, but rather a practical way in which most rail operators could easily improve safety at railway crossings today.
Related topics
Artificial Intelligence (AI), Digitalisation, Level Crossings, Safety, Security & Crime Management