Developing and deploying Automatic Train Operation in Russia
Posted: 10 March 2020 | Pavel Popov | No comments yet
Following recent projects and research into autonomous technology for trains in Russia, Technical Head of Development for Autonomous Trains at Russian Railways, Pavel Popov, explores how feasible an ATO future will be for shunting locomotives and commuter trains.
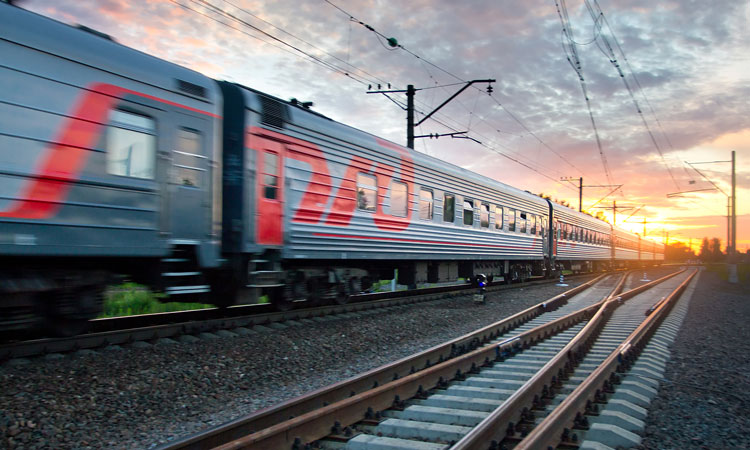
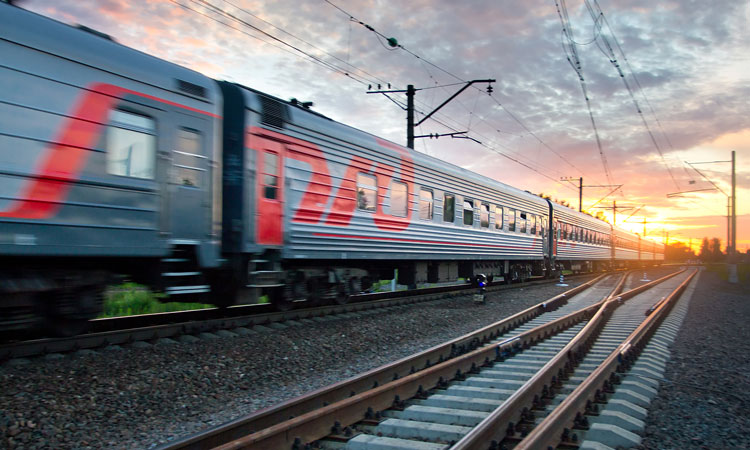
The history of Automatic Train Operation (ATO) started in 1957 in Russia when the first ATO system GoA2 was tested on commuter trains. Since that time, dozens of ATO systems have been developed for freight, passenger, and commuter trains, but it was only Level 2 according to IEC 62290. The vital step for the future railway is a transition from GoA2 to GoA3 and GoA4 that requires entirely new technology for a railway. The main challenge for GoA3 is to develop machine-vision, which works better than a driver. For the transition from GoA3 to GoA4, it is required to increase the reliability of the autonomous fleet and foresee all possible unusual situations and actions against them.
To find and work out a solution, Russian Railways launched two projects for autonomous vehicles.
Shunting locomotive
In 2017, the shunting locomotive TEM-534 was equipped with machine-vision sensors, new communication, and localisation equipment. The main objectives for this project were:
- To develop and test an obstacle detection unit
- To develop and test remote control.
Control of shunting work at the Luzskaya station is based on the shunting automated locomotive signalling system, called MALS. This system is similar to the RadioBlock centre. MALS has communication with the interlocking system and with shunting locomotives. Having information about routes, signals, the status of track circuits, and positions of shunting locomotives, MALS calculates movement authority for each vehicle and also speed profiles. The MALS automates a single closed-loop operation, which consists of next operation:
- Movement of shunting locomotive to the occupied track from the marshalling hill
- Coupling with wagons
- Pushing wagons on the marshalling hill.
These closed-loop operations repeat in autonomous mode many times per day.
In this project, we use machine-vision for obstacle detection and wagon detection for coupling. Our first shunting locomotive is used like our research laboratory to find the best sensors, computing units, localisation sensors and communication equipment. Next, there are subtasks for obstacle and wagon detection:
- To find the area of interest, so the region where machine vision has to detect obstacles and wagons. It means that it’s necessary to recognise the railway track ahead of our shunting locomotive
- To detect and track obstacles in the area of interest
- To calculate the braking curve for stopping in front of an obstacle
- To calculate the braking curve for coupling with wagons.
We collected data from our sensors under various weather and light conditions. Our conclusions for sensors for shunting locomotives are to use lidars, optical cameras with adding near-infrared range and stereovision. Current radars are not applicable for railway, due to clutters reflections.
During the first winter, we understood that it’s not enough to have only machine-vision for railway track recognition, because we met situations when our railway track was entirely under snow cover. Without the visibility of rails, it is not clear where to find obstacles or wagons.
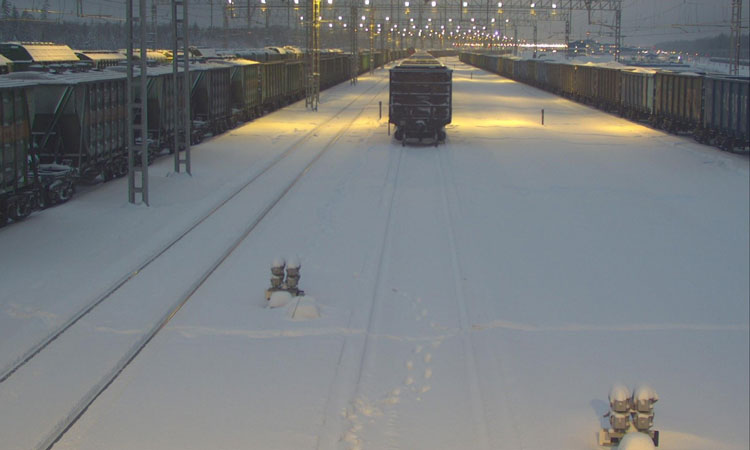
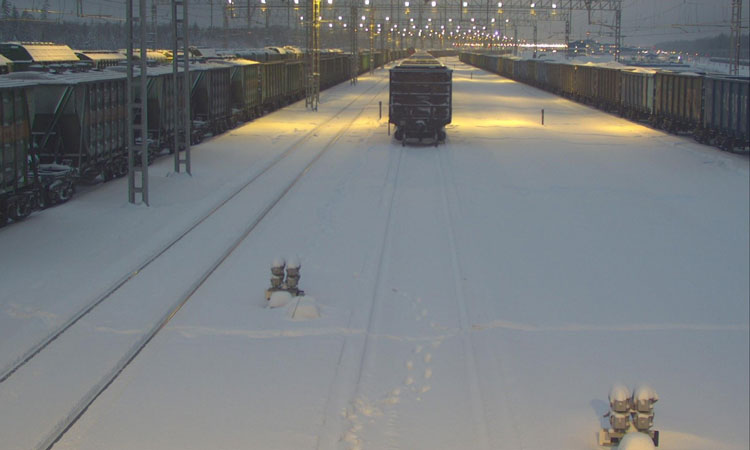
Data was collected from sensors under various weather and light conditions.
After that, we added a high precision on-board localisation system and digital map of the station. The excellent accuracy of localisation is provided by fusing different sensors, such as satellite navigation in RTK mode, an inertial navigation system, odometers, and different SLAMs algorithms (simultaneous localisation and mapping) based on perception. Even without snow conditions, combining machine-vision recognition of tracks and localisation with a digital map provides better results.
An important element to consider is how to ensure our obstacle detection system is working optimally, choosing between the probability of missing obstacles and the probability of false positives occurring. It’s a tradeoff. Our solution is to keep the probability of missing obstacles to a minimum (best variants is correspondence to SIL4 requirements). The downside of high-detection capabilities is an increase in the number of false positives. What is the necessary action in case of a false positive? Our solution is to use remote control, based on video streaming from a locomotive. At the Luzskaya station, we developed and tested the remote control with the possibility to monitor a few locomotives from one remote control place. The main challenge for remote control is to find a balance between the resolution of the video, frame per second, and the latency of the video.
To understand our progress, we have developed specific metrics for each subtask, which measures how well our autonomous locomotive works in autonomous mode.
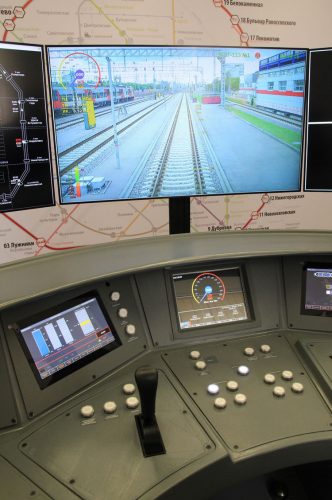
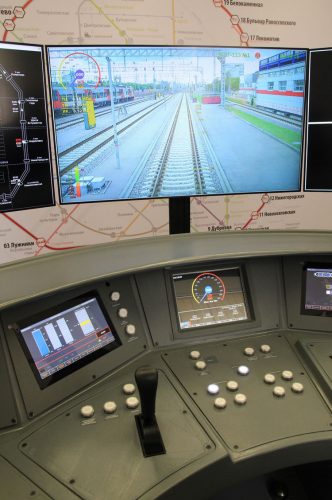
The main challenge for remote control is to find a balance between the resolution of the video, frame per second, and the latency of the video.
Commuter train
In 2019, we equipped a commuter train with machine-vision sensors and a high-precision localisation system. The self-driving commuter train is a much more complicated task, due to higher speeds and longer braking distances. We have significantly revised the choice of sensors and the use of algorithms. At the moment, we are actively testing our autonomous system for commuter trains. At the same time, we use similar approaches, like at the Luszskaya station. We also consider remote control as a crucial part of the self-driving trains.
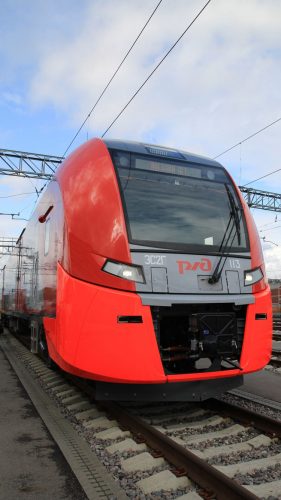
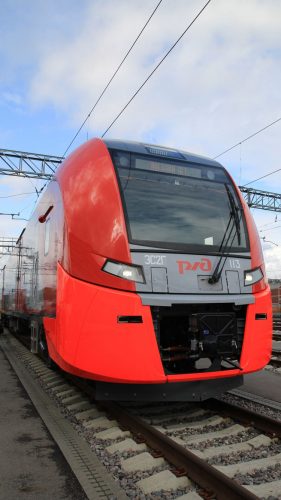
In 2019, Russian Railways equipped a commuter train with machine vision sensors and a high precision localisation system.
The key technology, which is widespread in the automotive industry, is a simulation of self-driving trains. There are a lot of automotive simulators for autonomous cars, such as CARLA, but nothing for autonomous trains. It’s not possible to test all conditions and all obstacles which can occur during operation by using on-site tests. For this reason, it’s mandatory to have a railway simulator for testing perception systems and other subsystems. We are currently developing a railway simulator and use it for some of our tests.
The current results of our projects showed us that autonomous technology for trains is feasible. The next questions which are necessary to resolve are safety cases and legislation.
The main problem is that current standards – such as EN 50126, EN 50128, and EN 50129 – do not have any information on how to prove safety for computer vision in different conditions. Our analyses showed that there is a new approach, which is called SOTIF (the safety of the intended functionality) and applied in the automotive industry. SOTIF is intended for autonomous vehicles and provides:
- Design measure example: requirement for sensor performance
- Verification measure example: Test cases with high coverage of scenarios
- Validation measure example: Simulations.
Our task is to develop the railway standard using the approach from SOTIF (ISO/PAS 21448:2019).
Regarding legislation, Russian Railways and the Russian Ministry of Transport developed a ‘road map’ for creating standards and documents which allow for the operation of self-driving trains.
Developing and deploying self-driving technologies promises to bring substantial economic benefits for Russian Railways in the future.
Related topics
Automatic Train Operation/Autonomous Train Control (ATO/ATC), Digitalisation, Operational Performance, Regulation & Legislation, Rolling Stock Orders/Developments, Technology & Software