The golden rules of AI in rail
Posted: 9 September 2024 | | No comments yet
InnoTrans, the major transport technology gathering, is just weeks away. As the sector gears up for this Berlin mega-event, Cohesive’s Andy Stephens considers the role of rail for one of the hottest technologies on the market: AI.
What’s the best approach, and what are the dos and don’ts for rail organisations hoping to maximise their value?
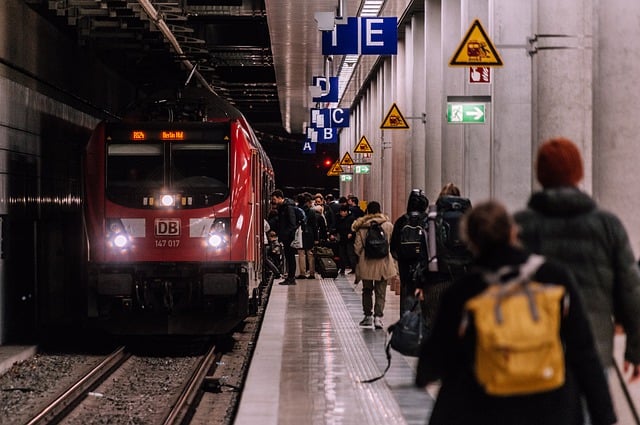
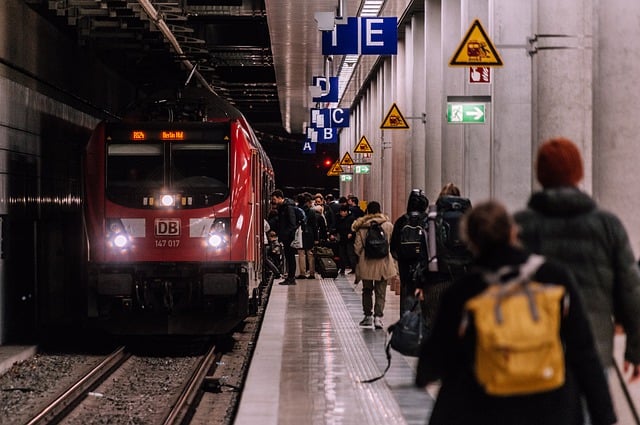
The rail sector is no stranger to hype. Exuberance by investors in 19th-century Britain sparked Railway Mania, which ranks among one of history’s biggest speculative bubbles. As the price of railway shares soared, speculators invested more money, which further increased the cost of railway shares until the share price collapsed and left many with hefty losses.
Today, the industry, like every other, is gripped by a hot new technology, which is generating fevered excitement: AI. Questions abound. How much to invest? How do we shape it to our needs? At what rate should we adopt it?
The answers to these will vary depending on the organisation. But one universal truth about AI in our sector stands: Its potential to generate value is completely linked to the quality of the data underpinning it.
Examples from global organisations, including Network Rail, show us what happens when high-quality data is not prioritised.
Network Rail has been using AI to help it move toward a ‘predict and prevent’ approach to maintenance rather than a reactive one. This means pre-emptively planning and fixing issues before they impact the railway and, consequently, journey times. AI algorithms analyse data from sensors installed on trains and tracks to predict equipment failures before they occur.
However, a study by the rail owner has highlighted that poor data quality from track sensors led to inaccurate maintenance predictions, resulting in unnecessary inspections and missed failures. That data quality can be improved through better sensor calibration and data cleaning processes.
Another example comes from the Massachusetts Bay Transportation Authority (MBTA). Like other rail organisations, it saw the potential of AI-driven systems to manage passenger flows in busy train stations. These systems rely on data from ticketing systems, surveillance cameras, and mobile devices to analyse passenger movements and optimise crowd management. The MBTA’s Railroad Operations Directorate, which manages the 5th largest commuter rail operation in the US with 500 trains per day on 14 different lines, implemented an AI-based passenger flow management system but faced challenges due to inconsistent and incomplete data from various sources.
Later this month, I’ll be in Berlin at InnoTrans, one of the most exciting events in the rail calendar. One of the key topics up for discussion there will be the use of generative AI in the sector and its likely impact. I am looking forward to sharing my knowledge and the stories and experiences of the rail organisations I work with and learning from others.
My advice is to follow the three golden rules to ensure the data you are feeding into AI systems is accurate, complete, and reliable. And compliance with these rules requires this:
- The establishment of robust data governance frameworks;
- Investment in data cleaning and integration tools; and
- Fostering within your teams a culture of data accuracy.
With these in place, rail can unlock the true potential of AI.
While AI holds immense promise for the rail industry, the importance of data quality cannot be overstated. High-quality data is the foundation for practical AI implementations, ensuring safety, reliability, and operational efficiency.
By following these rules, the sector can achieve AI’s true potential, deliver better services, and achieve sustainable competitive advantages in an increasingly digital world.
Andy Stephens has more than a decade of experience in the rail sector. He understands the power of technology and digital working to drive operational efficiencies, improve customer satisfaction, and enhance safety.